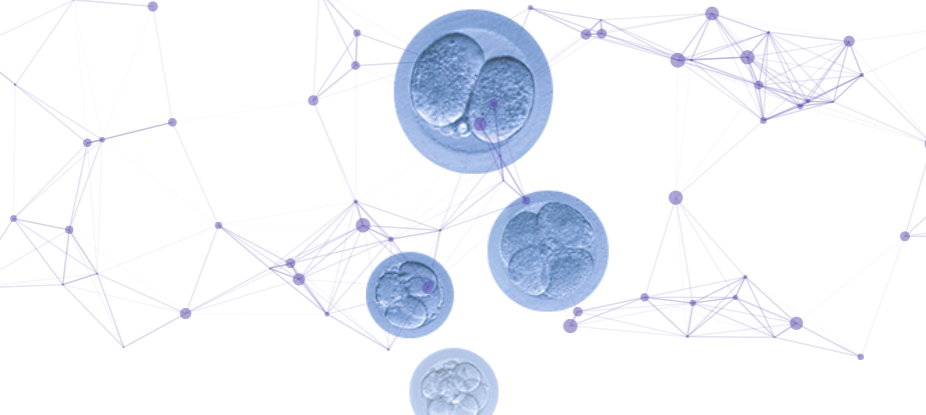
Can AI bring time-lapse imaging to the next level ?
by Yakup Yilmaz 30 May 2022
IVF success rates have remained relatively low for over a decade [1] while infertility is steadily becoming more of a burden to our society. To improve IVF success rates, a great deal of hope has been placed in new technologies & protocols to better diagnose and treat infertile patients such as Pre-implantation genetic testing, endometrial receptivity tests, or elective freeze all cycle [2]. Amongst these innovations, one has seen its generalization across clinics worldwide and particularly in Europe: the Time-Lapse Imaging system (TLI), which is at the heart of ImVitro’s first product
I: Time-Lapse monitoring: the controverse
- Environmental stability and better time management
TLIs are microscopes with a built-in incubator which allow the continuous monitoring of embryo development and thus, remove the need to take the embryos out of the incubator to visualize them as they develop. This conventional exit transiently disturbs the balance of the environment of the embryo, TLis are therefore ensuring optimal culture conditions.
Traditionally, embryo quality assessment is carried out to select the embryo to be transferred into the patient. It consists in evaluating morphological parameters of the embryo such as the number of blastomeres, blastomere size, nuclear status [3]. However, many criteria regarding embryo growth and development can’t be evaluated with static assessments, namely, pronuclei fading, time to cell division, abnormal cleavage or kinetics and these morphokinetic events have been known to be indicative of what embryologists deem being “good quality” embryos [4]
Time-lapse imaging has also brought a clear logistical benefit to embryologists. Indeed, TLIs eliminate the necessity of assessing embryos at fixed time intervals because the images of the embryos are recorded into a video, thus providing the flexibility of reviewing the developmental history at any time and facilitating the planning and timing of specific tasks in the lab.
- Impact on success rates?
But TLIs also held the promise of doing more than helping with time management. On the one hand, they could improve success rates by improving culture conditions, while on the other they could improve success rates by facilitating embryo evaluation and thus selection. But as of today, some scientists are yet to be convinced that TLIs do more than facilitate time management in the lab, and have one crucial question remaining : Do TLIs actually improve IVF success rates ?
According to the HFEA, ‘there is conflicting evidence from randomized controlled trials (RCTs) to show that it is effective at improving the chances of having a baby for most fertility patients’ [5]. “
A recent publication of a large RCT from 10 nordic centers involving 776 patients with at least 2 good quality blastocyst suggested TLIs and the morphokinetic annotations they facilitated might not affect success rates, showing an ongoing pregnancy rate for the time-lapse group of 47.4% vs. 48.1% in the control group with no statistically significant difference [6]. However, one major limitation to this study is that they only investigated the impact of TLI on the embryo selection of good quality blastocysts. What if evaluating morphokinetic events for embryos that seem of less good quality helped (especially if combined with artificial intelligence)?
Another major limitation of this study lies with the fact that only fresh transfers were included, which creates a bias. First, fresh transfers are almost exclusively reserved for patients that are normal responders or with primary ovarian insufficiency. Second, it is well known that transfer conditions are highly different between fresh and frozen embryos. Transfer success is dependent on a complex pre and post implantation dialogue between the embryo and the endometrium. [7] Frozen transfers offer a more controlled window of implantation, and therefore, transferring the best embryo for frozen transfers could reveal more clearly the impact of evaluating its morphokinetics. Moreover, according to ESHRE ¼ of the total number of transfer cycles in Europe are frozen embryo transfers (FET) and this share tends to increase considerably [8]. Studying success rates on fresh and frozen embryos seems thus necessary to draw conclusions on the impact of TLIs.
At any rate, such studies do suggest that the improvement of culture conditions alone brought by TLIs doesn’t seem to lead to greater success rates.
- Pondering the controverse : about the opportunities
The lack of evidence concerning the correlation between controlled culture conditions and improved success rates by TLIs has been weighted by high hopes in the emergence of deep learning models by many [9]. Indeed, the previously mentioned study only relied on human evaluation of morphokinetics. What if AI could surpass the way we assess embryos today, not only to save time but also to better predict the chances an embryo has to lead to pregnancy ? Further advancements in artificial intelligence applied to IVF are also likely to happen not only for embryo evaluation but also for gamete selection and treatment regimen selection. The massive generation of digital data gathered by TLI has now paved the way for artificial intelligence to bring IVF to the next level.
II: Bringing TLI to the next level
- Definition of AI
Artificial intelligence (AI), machine learning (ML), and deep learning (DL) can be confusing terms. To explore their applications in the IVF lab and more generally in healthcare, we have to define them first. AI encompasses ML and DL and describes algorithms that aim to mimic human or animal cognitive capacities. Machine learning, a subset of AI, describes techniques that give computers the ability to learn from data without being explicitly programmed. Finally, deep learning, a subset of ML, utilizes deep neural networks to learn from data. At its simplest, deep neural networks are layers of nodes, similar to the adjustments of neurons in our brain where each node is connected to another via multiple layers. The numerous layers allow models to become more efficient at learning complex features and performing more intensive tasks [10].
- Success of AI in healthcare
Deep learning applications in healthcare have recently increased tremendously, especially in medical imaging. Cancer diagnosis and radiology in particular have benefited from the emergence of unprecedented computing power and seen the development of AI based diagnostics tools. Seeing the overall exciting promises around deep learning application to healthcare begs the following question: does AI have its place in the IVF clinic ?
- AI for embryo evaluation
TLIs have been assisting scientists in the lab for more than a decade and its prevalence worldwide is expanding. A French national survey estimated that 40% of lab directors were TLIs users [11] in 2017. Now that these TLIs have become part of the IVF lab routine, numerous academic teams want to bring AI to the embryologist desk, but packaging it into a product that is CE marked, clinically validated across multiple clinics and maintained over time requires unique expertise
.
Indeed, implementation of AI in the embryo grading and selection process offers the opportunity to provide an objective, fast and accurate prediction of pregnancy and live birth outcomes. At ImVitro we however are not here to automate what the doctors can already do only to save time, we are here to surpass their capacity in predicting which embryo has the highest chance of leading to a pregnancy: this is a harder problem but one that will be a game-changer to increase success rates.
Embryo evaluation being arguably one of the most crucial steps of the IVF journey, different strategies have been adopted to perform it using AI. In 2019, Tran et al. published the first paper in the effort of predicting the probability of clinical pregnancy from time-lapse videos with deep-learning [12]. However, the use of videos alone has been of major concern to specialists since it neglects the clinical context of each patient and prevents the generalization of the algorithm to a variety of patients & IVF treatment protocols. Additionally, in Tran’s publication, numerous embryos that were discarded by the embryologist were assumed to never be able to lead to a pregnancy and used to train the algorithm even though they were never transferred, thus automating the bias of the embryologists [13]. On the other hand, Ver milyea et al, developed a model based on static images of D5 blastocysts and showed promising improvements in the accuracy of predicting clinical pregnancy [14]. Nevertheless, as discussed earlier, morphokinetics are known to be predictive parameters correlated with embryo implantation and these could be missed if only one image is analyzed [15].
Most recently, Jørgen Berntsen et al. tested their deep learning algorithm trained on videos of developing embryos in a variety of conditions such as patients age, insemination method, length of incubation and transfer protocol to investigate their performances in different clinical contexts. Overall, their performances were similar within the different subgroups (AUC ~0.67) except for cryopreserved embryos where they performed significantly worse [16]. However, these variables were not used to personalize the prediction – only the videos were analyzed. In the end, one issue persists, the generalizability of algorithm predictions to different clinical situations.
Conclusions:
The potential implementation of AI driven technologies in the IVF clinics has engendered enthusiasm and wide traction in the academic field, and even in industry with a few companies entering the field
Likely to be more objective, rapid, and accurate, deep learning applied to embryo evaluation has shown great promise towards improved IVF outcomes. However, to this date, existing algorithms have failed to demonstrate their generalizability to the variety of clinical contexts defining patients.
At ImVitro, we understand that there is no one size fits all tool to answer IVF needs.
Our tool is able to reliably contextualize embryo evaluations for different clinical characteristics and demonstrates a higher selection and deselection power depending on the clinical situation that embryologists find themselves in. Please contact us for more information about our performances, or come listen to our oral presentation in the 05/07/22 at 11:00 in Gold at ESHRE in Milan, or come meet us at our booth J30 !
References :
[2] https://www.hfea.gov.uk/treatments/treatment-add-ons/
[3] Alpha Scientists in Reproductive Medicine and ESHRE Special Interest Group of Embryology. The Istanbul consensus workshop on embryo assessment: proceedings of an expert meeting. Hum Reprod. 2011;26(6):1270-1283. doi:10.1093/humrep/der037
[4] David K. Gardner, Basak Balaban, Assessment of human embryo development using morphological criteria in an era of time-lapse, algorithms and ‘OMICS’: is looking good still important?, Molecular Human Reproduction, Volume 22, Issue 10, October 2016, Pages 704–718, https://doi.org/10.1093/molehr/gaw057
[5] https://www.hfea.gov.uk/treatments/treatment-add-ons/time-lapse-imaging/
[6] Ahlström A, Lundin K, Lind AK, et al. A double-blind randomized controlled trial investigating a time-lapse algorithm for selecting Day 5 blastocysts for transfer. Hum Reprod. 2022;37(4):708-717. doi:10.1093/humrep/deac020
[7] Jemma Evans, Natalie J. Hannan, Tracey A. Edgell, Beverley J. Vollenhoven, Peter J. Lutjen, Tiki Osianlis, Lois A. Salamonsen, Luk J.F. Rombauts, Fresh versus frozen embryo transfer: backing clinical decisions with scientific and clinical evidence, Human Reproduction Update, Volume 20, Issue 6, November/December 2014, Pages 808–821, https://doi.org/10.1093/humupd/dmu027
[8] European IVF-Monitoring Consortium (EIM) for the European Society of Human Reproduction and Embryology (ESHRE) , Wyns C, De Geyter C, et al. ART in Europe, 2017: results generated from European registries by ESHRE. Hum Reprod Open. 2021;2021(3):hoab026. Published 2021 Aug 5. doi:10.1093/hropen/hoab026
[9] https://www.focusonreproduction.eu/article/News-in-Reproduction-Time-lapse-monitoring
[10] https://www.width.ai/post/advantages-of-deep-learning
[11] Boueilh T, Reignier A, Barriere P, Freour T. Time-lapse imaging systems in IVF laboratories: a French national survey. J Assist Reprod Genet. 2018;35(12):2181-2186. doi:10.1007/s10815-018-1302-6
[12] Tran D, Cooke S, Illingworth PJ, Gardner DK. Deep learning as a predictive tool for fetal heart pregnancy following time-lapse incubation and blastocyst transfer. Hum Reprod. 2019;34(6):1011-1018. doi:10.1093/humrep/dez064
[13] A Chavez-Badiola, G Mendizabal-Ruiz, A Flores-Saiffe Farias, R Garcia-Sanchez, Andrew J Drakeley, Deep learning as a predictive tool for fetal heart pregnancy following time-lapse incubation and blastocyst transfer, Human Reproduction, Volume 35, Issue 2, February 2020, Page 482, https://doi.org/10.1093/humrep/dez263
[14] VerMilyea M, Hall JMM, Diakiw SM, et al. Development of an artificial intelligence-based assessment model for prediction of embryo viability using static images captured by optical light microscopy during IVF. Hum Reprod. 2020;35(4):770-784. doi:10.1093/humrep/deaa013
[15] Meseguer M, Herrero J, Tejera A, Hilligsøe KM, Ramsing NB, Remohí J. The use of morphokinetics as a predictor of embryo implantation. Hum Reprod. 2011;26(10):2658-2671. doi:10.1093/humrep/der256
[16] Berntsen J, Rimestad J, Lassen JT, Tran D, Kragh MF. Robust and generalizable embryo selection based on artificial intelligence and time-lapse image sequences. PLoS One. 2022;17(2):e0262661. Published 2022 Feb 2. doi:10.1371/journal.pone.0262661o